Design for Data Privacy – Keeping Data Safe and Secure
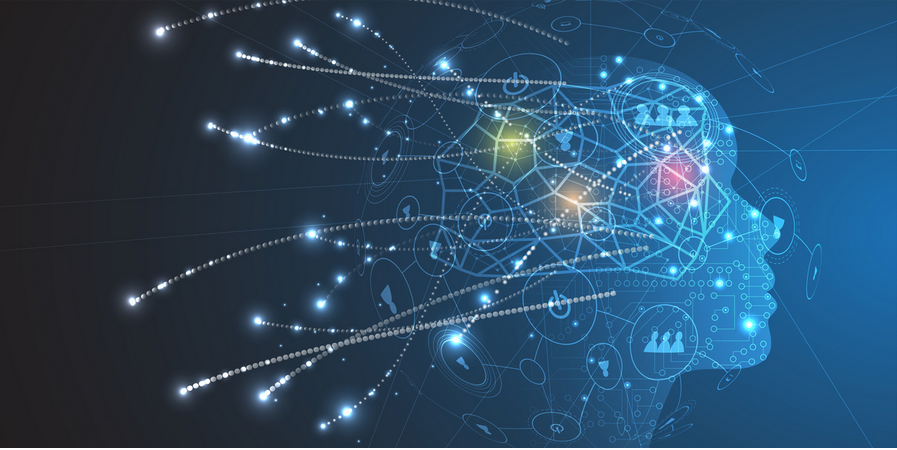
Keeping data private depends primarily on two factors. The first factor is the implementation of a security model, like the layered approach illustrated in Figure 8.1. Implementing a security model has the greatest probability of preventing malicious behaviors and bad actors from having an impact on your data and your IT solution in general. The tools and features that can help prevent those scenarios include VNets, private endpoints, Azure Active Directory, RBAC, and Azure threat protection functionality. The second factor has to do with the classification and labelling of the data, which needs to be kept private and requires an additional level of protection. A very helpful tool is linked to your SQL pools and other Azure SQL products: Data Discovery & Classification. As shown in Figure 8.10, when Data Discovery & Classification. is selected, the database is analyzed for content that might benefit with some additional form of classification and sensitivity labeling.
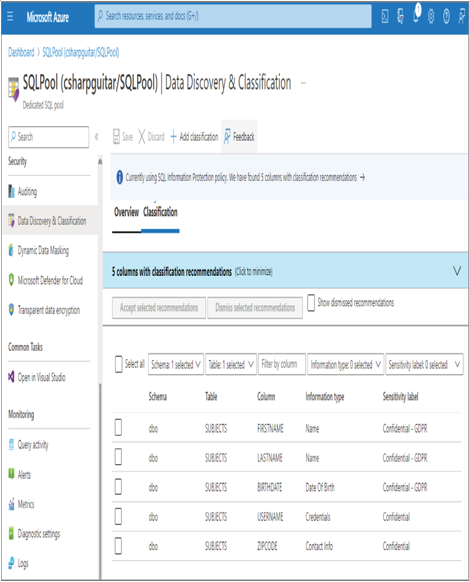
FIGURE 8.10 Data Discovery & Classification
Notice that selecting the Data Discovery & Classification navigation menu item resulted in a scanning of the dbo schema on the Azure Synapse Analytics dedicated SQL Pool. A table named SUBJECTS was discovered to potentially contain PII data, and the tool recommended the sensitivity label and the classification of the data in the Information Type column. The SUBJECTS schema is located on GitHub in the Chapter08 directory. Recognize that you are the expert when it comes to the data hosted on your database. Information stored in columns may be named in a way that results in it not being recognized as requiring additional protection. For this scenario, you can use the + Add Classification menu button to add both the classification and sensitivity label. When you select the + Add Classification menu button, the pop‐up window in Figure 8.11 is rendered.
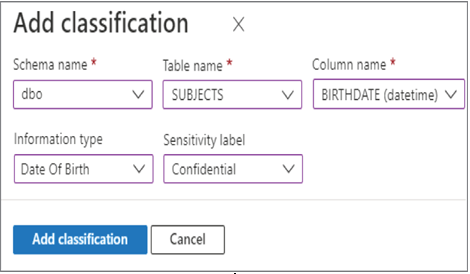
FIGURE 8.11 Data Discovery & Classification, Add Classification window
This feature exposes all the schemas, tables, and columns that exist on the database. After selecting add classification, you can then set the classification and sensitivity labels. In this case, the SUBJECTS table contains the column named BIRTHDATE and is classified as Date Of Birth related. The Sensitivity Label drop‐down is set to Confidential, which means that some kind of protective measure should be taken to control who can access the data. The other sensitivity levels are Public, General, and Highly Confidential. Reading through those examples, you can conclude that a sensitivity level of Public would likely not need any masking or encryption. As you progress through those different levels, the amount of security also increases with the maximum level of auditing, encryption, and access restrictions applied to the dataset as the Highly Confidential sensitivity level.
Data privacy also has to do with compliance regulations. There are many scenarios where data that is captured in one country cannot be stored outside the boundaries of that country. Data residency is an important point to consider when you are configuring redundancies into your data analytics solution. It is very common on Azure that datacenters are paired together and act as a disaster recovery option in case of catastrophic events. By default, for example, the West Europe region’s paired region is North Europe, and both are physically located in different countries. If your scenario requires that your data must remain in the country where it is collected in order to be compliant with your industry regulations, then take those appropriate actions. This capability is built into the platform for many Azure products, and when paired regions are located in different countries, you will be presented the option to disable this.